Unlocking the Potential of Image Labeling
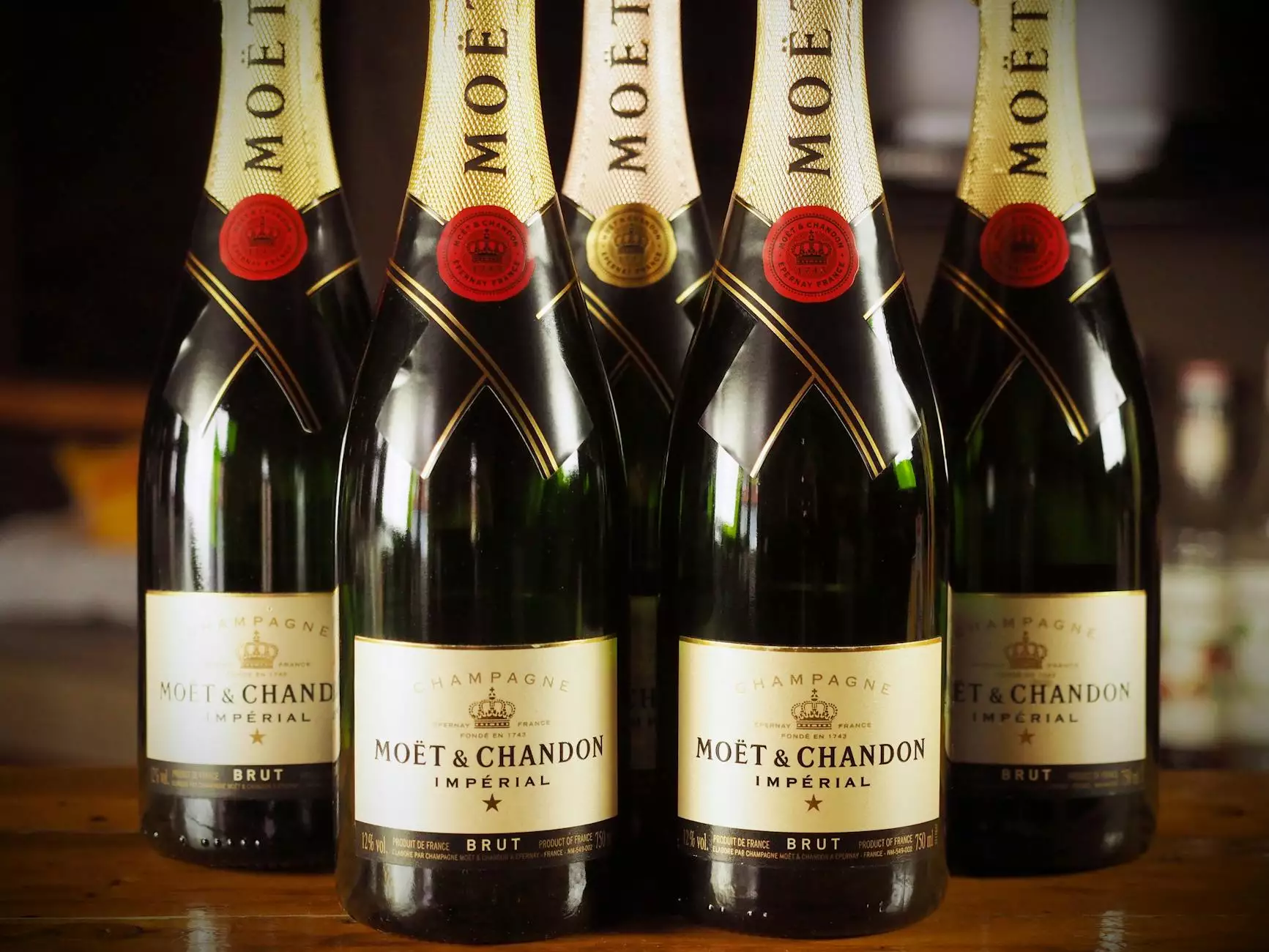
What is Image Labeling?
Image labeling is a critical process in the world of artificial intelligence (AI) and machine learning (ML). It serves as the foundational step in training AI models to recognize and interpret visual data. By applying labels or tags to images, we can provide machines with the necessary context to understand what they are analyzing.
The significance of image labeling extends to various applications, from autonomous vehicles interpreting road signs to sophisticated medical imaging technologies that assist in diagnosing diseases.
The Importance of Image Labeling in AI
In recent years, the use of image labeling has become paramount in the development of effective AI systems. Here’s why:
- Data Quality: Quality labeled data is crucial for training robust AI models. The model's accuracy is directly correlated with the quality and quantity of the labeled data it receives.
- Enhanced Learning: Through image labeling, machines learn to distinguish between various features and classes, which leads to improved predictive performance.
- Versatility: Image labeling can be utilized across many domains, including healthcare, security, retail, and beyond.
Types of Image Labeling
Understanding the different types of image labeling is essential for selecting the right method for your project. Here are the main types:
- Bounding Box Annotation: This is often the simplest form of labeling that places rectangular boxes around objects within an image.
- Polygon Annotation: This method offers more precision by allowing users to outline irregular objects with a polygon shape.
- Semantic Segmentation: This involves classifying each pixel in the image into a category, giving detailed insights about the visual content.
- Instance Segmentation: Similar to semantic segmentation, but it differentiates between distinct objects within the same class.
Challenges in Image Labeling
While image labeling plays a crucial role in AI development, it comes with its own set of challenges. Some of these include:
- Time Consumption: Labeling a vast dataset can be a tedious and time-consuming process if done manually.
- Subjectivity: Different labelers may interpret labeling requirements differently, leading to inconsistencies.
- Complex Scenarios: Labeling can become complicated with overlapping objects, occlusions, or varying lighting conditions.
KeyLabs.AI: Your Data Annotation Partner
At KeyLabs.AI, we recognize the nuances and challenges of image labeling. Our Data Annotation Tool offers a user-friendly interface, cutting-edge technology, and scalability to handle projects of any size.
We provide various annotation types to meet your specific needs:
- Customizable Workflows: Tailor your labeling process to fit the unique requirements of your project.
- AI-Assisted Labeling: Utilize our state-of-the-art machine learning algorithms to expedite the labeling process and maintain accuracy.
- Dedicated Support: Our team of experts is always available to assist with queries and provide ongoing support.
How Image Labeling Transforms Industries
The impact of image labeling transcends individual projects and spills into entire industries. Here’s how:
Healthcare
In healthcare, accurate image labeling allows for better diagnostic tools. Labeled medical images can assist radiologists in identifying anomalies, leading to faster and more accurate patient care.
Autonomous Vehicles
For self-driving cars, image labeling is vital for teaching machines how to interpret their surroundings. By labeling road signs, pedestrians, and obstacles, vehicles can make safe navigation choices.
Retail
In the retail sector, image labeling helps in identifying products in images for recommendations and inventory management, enhancing the overall shopping experience.
Best Practices for Image Labeling
To achieve the best results in your image labeling projects, consider these best practices:
- Define Clear Guidelines: Establish clear labeling guidelines to maintain consistency and quality across all labeled data.
- Quality Control: Implement quality control measures to review labeled data for errors and discrepancies.
- Use the Right Tools: Leverage advanced data annotation platforms to streamline your image labeling tasks.
The Future of Image Labeling
The future of image labeling is bright, with advancements in AI and machine learning paving new avenues for efficiency and accuracy. As technology evolves, we anticipate:
- Increased Automation: The role of automated tools will grow, reducing the need for manual labeling and speeding up the process.
- Enhanced Collaboration: Cloud-based platforms will facilitate real-time collaboration among teams, regardless of geographical boundaries.
- Greater Customization: Expect more tailored solutions to meet specific industry needs, thereby improving the quality of data annotation.
Conclusion
In conclusion, image labeling stands as a fundamental element in the realm of data annotation, shaping the future of AI and various industries. Leveraging platforms like KeyLabs.AI enhances your ability to annotate data efficiently and accurately, thereby unlocking the potential of your machine learning initiatives. Embrace the power of image labeling and position your business at the forefront of innovation.